Collective intelligence is a concept that generally refers to the knowledge that emerges from a group of people after a process of deliberation. For Levy, the concept arises in culture, which creates an environment in which human beings can select and reproduce ideas. Political scientists, such as Habermas, have also linked this concept to political decision-making, arguing that the process of deliberation leads to the creation of new societal solutions.
Naturally, there are several considerations embedded in the concept of collective intelligence. First, the “collective”, which involves understanding who participates in this idea generation and to what extent their original thoughts and values are representative of the group that they belong to. Second, the “intelligence”, which involves understanding the relevance of the ideas and decisions that emanate from the collective through the process of deliberation.
Over time, researchers have identified values that promote the development of collective intelligence, such as inclusion and respect, along with attributes, such as the presence of a moderator, or the use of expert advice. As such, many Internet or digital tools have been used to foster collective intelligence, from deliberative forums or platforms to social media, to collaborative tools. A well-known example of collective intelligence in action is found in Wikipedia, a collaborative online encyclopedia that was written by millions of people globally and is often found to be more relevant and useful than traditional encyclopedias written by a small group of people.
What happens, however, if all or most of the members of the collective have similar values and ideas, and if those with different perspectives are unable to participate? Similarly, what happens if the collective is so large that any new ideas are immediately diluted, and unable to move the needle? These are questions that have also emerged in another “intelligence” process, that of artificial intelligence.
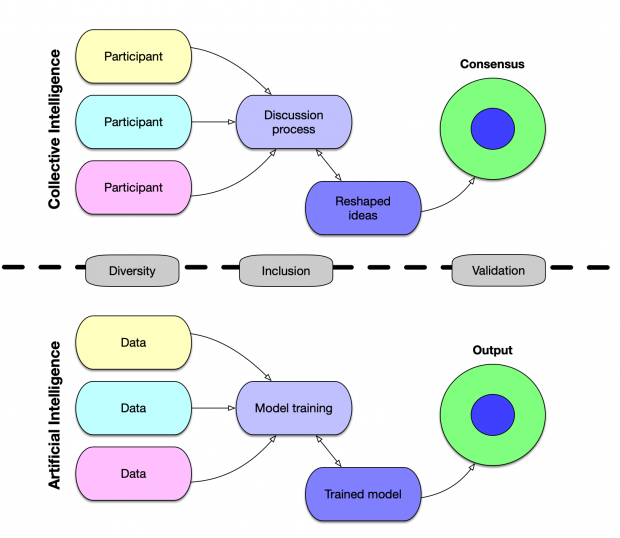
Artificial intelligence has so many similarities with collective intelligence that it might be mistaken as such. Algorithms gather data, which may be akin to individual persons’ contributions, and build models which will provide the most correct outputs based on the input data. Recently, these models have been used to generate text, which represents, in a way, a convergence of ideas based on a variety of inputs.
One of the best-known AI models used currently in text generation is called GPT-3, which is used in a wide variety of applications from chatbots, to translation, to blog writing. It has even been used to write Wikipedia entries, which, on the surface, appear quite convincing.
However, from a gender perspective, there are several concerning failures of such algorithms to lead to real collective intelligence. In other words, the text generated by GPT-3 can be biased against women, in that it propagates harmful stereotypes against women. And it is not the only model to do so. Gender stereotyping in natural language models has been extensively documented. In practice, it means that that the models will write about women as homemakers more often than they will write about them being leaders and programmers. It also means that chatbots can write sexist commentary, and even in some cases, show outright violence towards women.
GPT-3 has also been shown to perpetuate gender stereotypes in AI-generated stories. Notably, when used to write short story texts, the model chose to feature male characters more often, and when it featured female characters, it associated them with family, emotions, and body parts, rather than politics, sports, war and crime.
But why does this happen? First, there is the training data. The table below shows the online sources used to train GPT-3.
Dataset |
Quantity of tokens |
Weight in training set |
Common crawl |
410 billion |
60% |
Web Text 2 |
19 billion |
22% |
Books 1 |
12 billion |
8% |
Books 2 |
55 billion |
8% |
Wikipedia |
3 billion |
3% |
The data used to train these models promotes stereotypical gender roles which downplay women’s political and economic participation in society. The Common Crawl data, which, as its name suggests, is a huge dataset of articles extracted from the Internet, is known to contain quite a bit of gender bias. WebText is a very similar data source, which was developed by Open AI also by crawling the web, although possibly with a different method. The source of books1 and books2, in fact, has not been released by Open AI. It is presumed that these are extracted from large book repositories, but it is unclear what books exactly.
Finally, there is Wikipedia. Although Wikipedia only represents 3% of the dataset used in GPT-3, it does warrant further discussion, particularly due to its use as an exemplary output of collective intelligence. 90% of contributors to Wikipedia, in fact, are male, according to a 2015 study by the Wikimedia foundation. This gender divide has certain consequences on the quality of female representation on Wikipedia. In fact, the study showed that the entries of female leaders and historical figures were less likely to be extensively documented on Wikipedia.
Biases in natural language processing models show one of the major risks in using artificial intelligence as a collective intelligence exercise. If they are not adjusted, these biases can influence the way we think about women and their role in society, and slow down progress towards gender equality.
But are we doomed to a vicious cycle of gender stereotypes? Thankfully not. For artificial intelligence, technical solutions have been proposed, such as retraining the models on smaller, unbiased datasets, or randomising pronoun selection (in translation algorithms). Other solutions include gender impact assessments of tools before they are deployed to the public, as well as continued investment in the representation of women in the AI sector. As for collective intelligence, issues such as the cultural and historical portrayal of women are complex. However, as we develop more and more AI tools, making sure that these contribute to the advancement of women in society, and not the other way around, will be critical.
In the blogs in this series, we will unpack some of the factors impinging on the achievement of collective intelligence within the United Nations, highlight the alignment of collective intelligence with some of the key principles of the United Nations, undertake detailed case studies of how collective intelligence is being operationalised and employed across some of the UN agencies, and share our work on leveraging digital technologies to enhance collective intelligence within the UN system for solving sustainable development challenges.
Suggested citation: Fournier-Tombs Eleonore ., "Addressing (Un)intelligence: Gender Stereotyping in Collective and Artificial Intelligence," UNU Macau (blog), 2022-09-13, https://unu.edu/macau/blog-post/addressing-unintelligence-gender-stereotyping-collective-and-artificial.