Artificial Intelligence's (AI) rise in the Fourth Industrial Revolution promises significant economic gains, yet raise ethical dilemmas, from data bias and misinformation to intellectual property concerns. Recognizing the urgency for ethical oversight, UNU-Macau, in collaboration with UNESCO and the University of Macau, organized the international workshop titled "Ethical AI: Pioneering Progress in the Asia-Pacific". The workshop aims to facilitate multi-stakeholder dialogue, uniting experts, policymakers, and stakeholders to explore the ethical dimensions of AI and seeks to catalyze collective efforts toward responsible AI development and deployment in the region. This article synthesizes insights from three keynote speakers of the workshop, exploring the latest trends in AI ethics from philosophical, legal, and business perspectives.
AI Ethics from the Ground Up: Toward a New Theory of AI Ethics
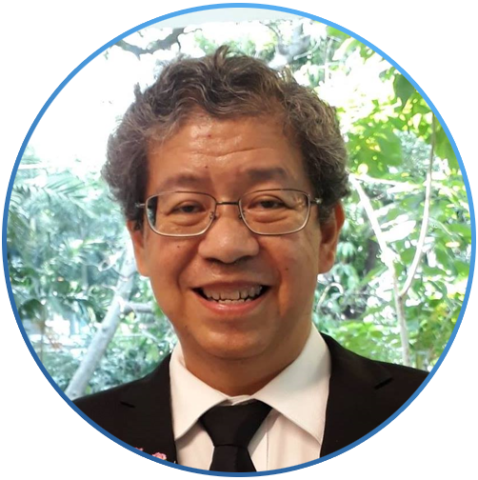
Soraj Hongladarom, Professor from Chulalongkorn University in Bangkok, starts to answer the question: why do we need a new theory on AI Ethics. The dominant theories in ethics are the deontological, the utilitarian, and the virtue ethics theory. But when it comes to an ethics of AI, these theories do not seem to be adequate in providing a satisfactory account of why we should have the guidelines that we have. For example, privacy is usually justified in terms of protection of individual rights, but rights often do conflict with one another. Many modern virtue ethics theories do not specify exactly what exactly is the good life that virtuous acts are supposed to bring about.
What we need, instead, is a theory that defines what it is for an AI system to be ‘good’ or ‘virtuous’ or ‘excellent’ – and his proposal is that we can start to come to know this through a realization that the normative is derived from the natural. The idea is very ancient, which can be found in Daoism, Buddhism, Stoicism, and so on. In Buddhist term, this means that the action does not originate from a selfish motive, but a selfless one.
One example is “Black Box AI”, which is a problem for many because by being unexplainable, the AI system generates lack of trust and even fear, even though it generates correct answers almost every time. But we don’t fully understand our own brain either – I am not able to explain how my brain works at the neuron level – but I can certainly explain why I think what I think, so AI system should do the same – that is, explanation at the level of justification or rationale but not the decision is made at the ground level. To do so would show that the AI system does follow nature because it creates trust and understanding.
Another example is biased algorithm. The biases usually come from the datasets themselves. So a way out should be to improve the quality of the data (which must go hand in hand with improvement of the quality of the algorithm too). Key ethical issue lies in the question whether certain situations count as being biased or not. Here the theory can help – we recognize social (cultural, racial, ethnic, etc) biases when the outcomes of the algorithm lead to furthering or deepening of the biases, rather than the other way round. These biases certainly comprise the unsatisfactory condition that the Buddhism-based theory aims at eliminating.
What would happen if one culture does not recognize the same total set of ethical values as another? This happens all the time in history – but not in the way that critics of relativism seem to think. The similarities in cultures’ ethical judgments are more to be found than the differences – honesty, compassion, caring, truthfulness, love, sympathy, etc. This can be seen also in the fact that most AI ethics guidelines share much in common – the big three principles are transparency, privacy, and algorithmic fairness.
Thus, when we try to construct a meta-theory of how values from various culture should form a basis for a set of universal values, we can start from the ground up. That is, from the fact of the natural conditions in each culture, rather than from pure a priori theorization. It is as if the workings of the AI system themselves provide a limit on how much ethical values in these cultures could diverge.
Artificial Intelligence and Sustainable Development: Arguments for Global Binding Regulation
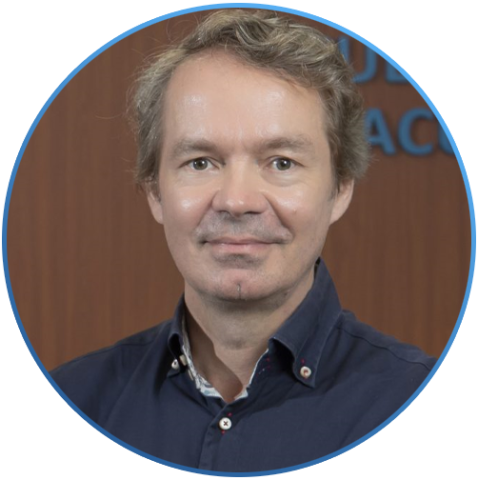
Rostam Neuwirth, Professor from University of Macau emphasizes that the relationship between ethics and law should be collaborative rather than competitive as the field advances. Currently, various countries and international entities, including China, the US, EU, OECD, UNESCO, and the UN, have introduced ethical documents on AI, creating a sense of competition for the 'Global Regulation of AI Governance.' It is necessary of complementary frameworks at the global level.
There are considerable contradictions within the term of AI and sustainable development. Some researcher said that from the perspective of psychology of intelligence, the term artificial intelligence is an oxymoron. Intelligence, by nature, cannot be artificial and its inestimable complexity defies any notion of artificiality (Jennifer Gidley, 2017). Similarly, researchers argue that “sustainable development,” as advocated by most natural, social, and environmental scientists, is an oxymoron. Continual population growth and economic development on a finite earth are biophysically impossible. They violate the laws of physics, especially thermodynamics, and the fundamental principles of biology (James Brown, 2015).
Over the past seven years, there has been a notable increase in the use of terms like Oxymora and Paradox across various disciplines, including law. This trend is intriguing, especially for someone like Neuwirth, who, being trained as a lawyer, was accustomed to distinguishing between what is legal and illegal, right and wrong, without room for simultaneous guilt and innocence. The discourse around contradictory terms, such as the Oxymoron of "soft law," has become prevalent. This term is often debated because, traditionally, if the law is soft, it's not perceived as law. The growing frequency of employing Paradox and Oxymora is also evident in proposals addressing the regulation of emerging technologies. As David Held said, the paradox of our times can be stated simply: the collective issues we must grapple with are increasingly global and, yet, the means for addressing these are national and local, weak and incomplete.
Recent research has delved into the impact of emerging technologies on the human mind, viewing the brain itself as a complex black box that is challenging to control. Neuwirth expressed concern over the potential ease with which someone else could manipulate the mind if even the individual cannot fully control it. Notably, studies, including one by Oliver Whang, indicate that AI is decoding aspects of brain activity. Some research successfully visualized images based on what a person had previously seen, sparking debates in law about the need for adaptations to address these rapid technological advancements. The right to freedom of thought serves as a pertinent example, prompting discussions on revisiting how we interpret such rights in the context of evolving technologies and laws.
AI serves not only as a creation and a tool but also as a crucial mirror reflecting the black box of our minds—revealing aspects that largely exist beyond or beneath our conscious awareness. Neuwirth emphasized the need to explore these hidden realms, suggesting that new technologies can enhance our understanding of the intricate workings of the mind. In light of this, he stressed the importance of shaping legal systems and thoughts to align with these advancements.
Towards Responsible and Verifiable AI: A Voice from Industries
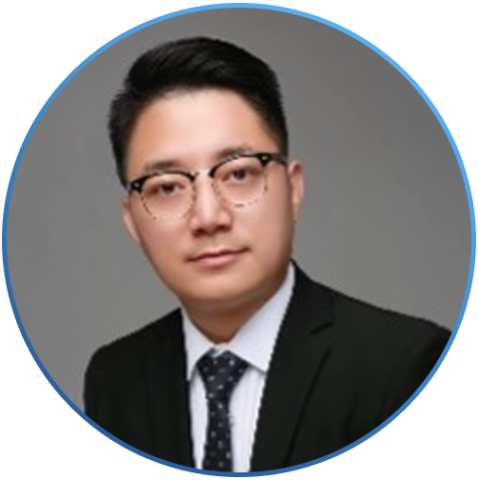
Zhengkun Hu, Director of AI Ethics and Governance at SenseTime, brings perspective from industries. AI has been around us for quite some time. It was not until the recent decades that we start to see some of the AI applications going into different industries. For example, the algorithms to keep our financial transaction safe, and also to help us to detect early stage of cancer and also assist us in driving our vehicles. And those are the type of applications that we had in the past ten years. But the appearance of ChatGPT is a game changer because it indicates that large language models, pre-trained models could be the way to lead to a more general intelligence and it represents a new paradigm in the development of AI.
The emergence of large language models like ChatGPT poses challenges to traditional AI governance models. Unlike models designed for specific tasks with clear objectives, large models are more versatile, serving general purposes. This versatility complicates the evaluation process, as the scope of assessment becomes unclear before applying these models to specific applications. The key challenge in AI governance now lies in evaluating and assessing the risks associated with large models due to their myriad potential applications. This shift has prompted a new wave of discussions and public discourse on AI governance globally. Hu emphasizes four points on AI governance, particularly from the standpoint of AI developers and providers:
- AI governance is an integral part of AI development, and will help society-wide adoption of AI. There exists a misconception that AI governance is separate from AI development, but in reality, issues like hallucinations in large language models pose governance challenges. AI governance is now an integral part of the overall AI development landscape.
- Establish an effective framework that can adequately address immediate, near-term, and long-term risks, which include ethical, social-economic, political, and existential risks. We must to differentiate risks so that to make our governance framework more effect and more poor innovation so that we don't put breaks too early in the early stage of AI development.
- Risk assessment and evaluation should be context-based. It's more practical to make risk assessments in the application context. We need to differentiate the application scenarios of AI, so that we can have a clear definition of the risk.
- Invest in AI governance infrastructure. When discussing AI governance, the focus often revolves around large dataset training and computing facilities. However, a comparable infrastructure is crucial for the successful implementation of an AI governance framework.
SenseTime's journey in operationalizing AI governance involves turning values into implementable standards, incorporating AI ethics into product design, and embedding ethics into day-to-day operations. Over the past two years, they have constructed internal governance infrastructure, addressing data processing to creativity. Additionally, SenseTime has established a technology alliance for sustainable development goals in collaboration with other industry partners.