Introduction
In the digital era, data reigns supreme. It’s the cornerstone of evidence-based decision-making and the foundation of transparency and accountability. With the race towards the 2030 Agenda, in the final leap, we’ve witnessed an unparalleled surge in data demand, sparking a revolution in data innovation. Data isn’t just about having numbers; it needs to be accurate, timely, detailed, relevant, and accessible - empowering decision-makers to act with confidence and clarity.
The demand for data-driven solutions has ushered in the era of Artificial Intelligence (AI)-powered data analytics, - transforming how we approach global challenges. AI-powered data analytics have been employed to enhance poverty mapping, enable precision agriculture techniques, improve healthcare diagnostics, and offer personalized education which significantly contribute to the Sustainable Development Goals (SDGs) Agenda.
The success of AI in achieving equitable outcomes hinges on the quality and availability of data. Yet, we face a critical challenge of a lack of gender-specific indicators, leading to what is known as the gender data gap. Several factors contribute to the existence of gender data gaps, including biases and discrimination that can occur during the processes of data collection, analysis, and interpretation. Addressing this gap is crucial for creating inclusive AI systems that understand and serve the needs of every individual. Yet, as highlighted in the Global Gender Gap Report of 2023, women represent only 30% of the workforce in AI, underscoring the critical need for diverse perspectives in technology development. As one insightful perspective puts it, "When technology is developed with just one perspective, it’s like looking at the world half-blind."
Synthetic data presents a promising solution for closing gender data gaps by supplementing real-world data when such data isn’t readily accessible. By addressing data scarcity issues, synthetic data offers opportunities to foster equitable AI development, aligning with the SDGs. However, as highlighted in a recent policy brief by the United Nations University, while synthetic data offers significant benefits, it also poses risks and challenges that could potentially amplify existing gender inequalities. In our era of accelerating digital transformation, the digital divide manifests not only as the lack of internet access but also as digital exclusion in datasets, leading to a lack of voice and representation especially for marginalised groups in the global south.
The Gender Data Gap
Despite global commitments to gender equality, the gender data gap remains. The gender data gap reflects the absence of data related to the differing experiences of women, men, girls, boys, transgender men and women, and non-binary individuals. Currently, only 48% of the data needed to track progress on SDG 5 is available. No country among the 193 committed to Agenda 2030 has complete gender-specific SDG data. At the current data growth rate of 3% annually, it will take 22 years to gather all the necessary SDG gender data - more than a decade past the 2030 deadline.
From a gender perspective, one of the most critical ethical considerations is AI’s potential to reinforce existing gender stereotypes in its recommendations and decision-making processes if not trained on datasets that address gender data gaps. This issue is already evident due to pervasive gender data gaps, which have led to significant intersectional gender inequalities. A UNESCO study reveals alarming evidence of regressive gender stereotypes within large language models. Women were described as working in domestic roles far more often than men - four times as often by one model - and were frequently associated with words like “home,” “family,” and “children,” while male names were linked to “business,” “executive,” “salary,” and “career.”
When it comes to another sector such as healthcare, biased datasets can skew AI predictive algorithms. Take gender bias, for example - AI tools used to screen liver disease were shown to work against women, leading to less accurate diagnoses. Then there is the race-based correction factor in kidney function tests for chronic kidney disease, which was found to potentially delay diagnosis and treatment for black patients. In other contexts, the use of AI in judicial sentencing has raised concerns over potential discrimination against black offenders. This adds another layer to the ongoing debate about fairness and bias in AI, highlighting the critical need for more equitable and transparent systems across all sectors.
At the current data growth rate of 3% annually, it will take 22 years to gather all the necessary SDG gender data - more than a decade past the 2030 deadline.
The Promise of Synthetic Data
Imagine a world where the biases and gaps in AI systems are a thing of the past. Sounds like a dream, right? Well, the field of synthetic data is making strides towards turning this dream into reality. By creating diverse and balanced datasets that mirror the real-world population, synthetic data is helping us build AI systems that are fair and representative. But you might be wondering, what exactly is synthetic data? And how can it be harnessed to bridge the gender data gaps?
Synthetic data refers to data that is artificially generated in the digital world and often possesses characteristics inherited from an ‘original’ dataset. This contrasts with real-world data, which, as the name suggests, is data collected from real-world events and inputs. Synthetic data can be used as an alternative or supplement to real-world data when real-world data is not readily available. Synthetic data can be simulated in such a way as to have many of the same properties as the original dataset, and to allow derivation of the same results and insights, but with a much lower risk of revealing information about individuals to which that data relates. Synthetic data are increasingly being used to train AI algorithms, especially when real data is sensitive, scarce, or biased.
Closing gender gaps in data is a crucial step towards achieving equity, and synthetic data is emerging as a game-changer in this effort. By providing balanced datasets, synthetic data ensures AI models deliver fairer and more equitable outcomes. Synthetic data enriches small datasets, safeguards privacy, democratises data access, and addresses ingrained biases, making it an economical and adaptable tool for fostering an inclusive digital landscape.
For instance, in healthcare, synthetic data protects patients’ privacy and enhances clinical trials by offering broader research access and realistic data for software development and testing--all while maintaining anonymity. In education, synthetic data helps improve machine learning models, such as SDG classifiers, ensuring they are trained on diverse and representative datasets. Additionally, National Statistical Offices (NSOs) can use synthetic data to solve statistical disclosure problems, enabling more accurate and inclusive data analysis.
Harnessing the power of synthetic data comes with its own set of challenges and opportunities. For organisations looking to train AI systems with synthetic data, the key to reaping the benefits lies in their ability to mitigate associated risks. This ensures that the use of AI remains aligned with legal standards and ethical principles. The risks of utilising synthetic data are multifaceted, ranging from cybersecurity threats to the perpetuation of biases and an escalation in model inaccuracies. Issues such as data integrity, misuse, intellectual property (IP) infringement,data pollution,and contamination also loom large.
Additionally, synthetic data can create false confidence in dataset diversity and representation, as seen in facial recognition technology evaluations. Additionally, synthetic data can bypass the need for consent in data usage, complicating governance and ethical practices by decoupling data from the individuals it represents. While beneficial for training AI and addressing gender gaps, synthetic data isn’t perfect. AI models trained on biased datasets can perpetuate these biases, especially in healthcare, leading to higher error rates and misdiagnoses for underrepresented genders. Overlooking socioeconomic factors can further entrench inequities. The success of synthetic data hinges on ethical, diverse, and representative practices to ensure it benefits everyone without perpetuating stereotypes or biases.
Addressing these concerns, the United Nations University has put forth the “Recommendations on the Use of Synthetic Data to Train AI Models.” These guidelines offer concrete measures to facilitate the ethical and responsible employment of synthetic data in AI development. Adherence to these recommendations could empower organisations to not only navigate the complexities of synthetic data but also to leverage it as a tool for bridging gender data disparities. The goal is to achieve this in a manner that is safe, equitable, and precise, paving the way for more inclusive AI solutions.
Conclusion
In conclusion, synthetic data holds significant promise for bridging gender data gaps by supplementing real-world data, thereby fostering more equitable AI systems and supporting global gender equality efforts. To achieve this, it is essential to involve focused research to assess the risks and benefits of synthetic data on gender data gaps to determine its effectiveness and address ethical concerns, such as privacy and the potential reinforcement of stereotypes.
Additionally, research should prioritise the development of robust synthetic data generation methods that accurately represent diverse gender identities. Such efforts should also include the creation of rigorous testing and validation frameworks that assess the accuracy and fairness of AI models trained on synthetic data, with benchmarks for performance across different genders.
For policy, this means implementing guidelines that ensure the ethical and equitable use of synthetic data, ultimately supporting the SDGs by fostering gender equality and reducing disparities. The benefits include informed decision-making, enhanced monitoring, inclusive policy design, accelerated progress towards SDGs, and risk mitigation.
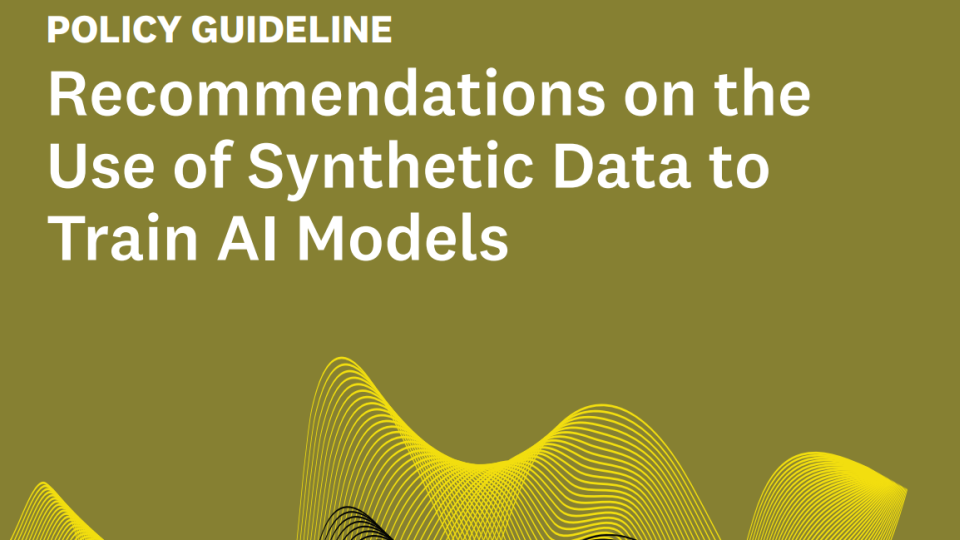
Suggested citation: Musizvingoza Ronald., "Bridging the Gender Data Gap: Harnessing Synthetic Data for Inclusive AI," UNU Macau (blog), 2024-08-02, 2024, https://unu.edu/macau/blog-post/bridging-gender-data-gap-harnessing-synthetic-data-inclusive-ai.