by Franz Gatzweiler1, Cara Antonaccio1, Chokdee Rutirasiri2
1 United Nations University – Institute in Macau
2 The Broad Institute of MIT and Havard
After many years of experience in participatory resource management, conservation and public health projects, we recently had the privilege to observe the implementation of a computational participatory modelling exercise with the goal of building collective intelligence to optimize the management of resources in health facilities and communities. The stated objective of this exercise was to more efficiently and effectively (in terms of resource allocation) respond to an outbreak of an infectious disease. Being a complex albeit tenuously defined problem, this exercise was intended to address the problem by engaging stakeholders in a process which would eventually lead to building a computerized (agent-based) model.
What became evident during the process of engaging stakeholders in a participatory modelling exercise, was that the facilitators attach some value to the human subjects they refer to as stakeholders; that the people who participate in the modelling exercise are reduced to agents, who themselves become a source for behavioral data, and that despite being referred to as ‘citizens’ (in a citizen science research) are not part of a community building process, which allows the community to define their own shared problem.
Seeing people as a resource for behavioral data, means attaching utilitarian values to people. According to this utilitarian worldview, the process of obtaining the most and best data from participants will enable researchers to build models which reflect their realities, even if these models are predicated on a series of potentially problematic assumptions, which establish the computational model’s set of few, rather arbitrary and reductionist rules. A problematic assumption is, e.g., utilitarian and individual rationality of stakeholders in a humanitarian context, when participants would apply social rationality (when money doesn’t matter).
The design of the research process that follows that ‘efficiency’ approach separates the collection of data from building knowledge (e.g., building and running the models, running simulations and building scenarios) and making decisions on actions which are supposed to be achieved through a scientific process. In the learning cycle of this approach, all components of the learning cycle are carried out in isolation: data, knowledge, action and evaluation. This research design by dissection may lead to knowledge gains on the side of the researcher, but it does not lead to collective intelligence gains for and empowerment of the participating stakeholders.
This approach, referred to as “participatory”, engages stakeholders for the sake of their value as data deliverers; behavioral data and data about resource flows which reduces citizens to agents and their complex realities to a simple set of behavioral rules. While all models are necessarily a simplification of reality, some simplifications are done to fit the model while others attempt to representing participants’ reality, build trust and empower them. Scientists who are under pressure to demonstrate excellence, to come up with innovative interdisciplinary methods and to publish, can be tempted to apply this approach without realizing the type of utilitarian values they attach to people, who become their human subjects. Sloane et al. (2020) have drawn attention to the problem of using participation as a design fix for machine learning and differentiate between different qualities of participation as work, consultation and justice. They conclude that “given the extractive and oppressive capitalist logics and contexts of ML [Machine Learning] systems, it appears impossible to design ML products that are genuinely “just” and “equitable”.
This is an all-too-common problem that continues to impact and marginalize, and in some situations, may even bring additional harm to the communities being studied. While a participatory model seeks to develop a deeper, more holistic understanding of a community, e.g., behavior, values, well-being, safety, culture, etc. it does not engage long enough to empower the community members to play a greater role in decision -making and interventions that have direct impact on their lives – something which is aimed for in post-normal science and transdisciplinary approaches.[i] , [ii]
A basic participatory model may be a good start, but its lens is too often limited by researchers and facilitators who are left to synthesize, interpret, and frame factors that impact communities, usually, which they are not a member of themselves; they are outsides looking in, yet their social impact could be equal to or greater than the actual people living in the communities they are studying.
In particular, we raise concerns when the stated purpose of participatory modeling is to build trust by means of a participatory computational model but when the goal of a project is driven by the attempt to demonstrate how models can be built and what their strengths might be, without proper appraisal of their inherent limitations. When such a shift in focus occurs, the involvement of people becomes merely a means to an end of demonstrating the capabilities of computerized models and people are merely there to elicit data from in the participatory process, in order to deliver data for the computer models. If those models are agent-based, they aim at simulating the actions of individuals or organizations (agents), aiming to explain collective behavior of agents, who behave according to a set of simple rules. The individual agents are typically assumed to be boundedly rational and self-interested. Once the agents’ behavior is defined, simulations can be run to test how changes in individual behavior affect the entire system. While communication and social behavior are usually too complex to be assigned to the behavioral properties of the agents, agent-based models do show the phenomenon of emergence – novel behavior of the system, which cannot be explained by that of its parts.
The technical part of building and training the model is done separately by computer scientists or mathematical modelers. This is often justified by arguing that people are not sufficiently literate to build and operate computerized models. Obviously, there is a large amount of risk implicit in this mindset where researchers may become wedded to a model or set of restrictive rules or assumptions. Not only can these models be a poor representation of people’s “reality”, their haphazard construction itself can cause unintended harm to humans participating in the exercise.
An approach that attaches intrinsic value to people, in contrast, recognizes that humans have value in and of themselves through their lived experiences, independent from being useful as data deliverers based on the preconceived notions and expectations of outside observers. The aim of computerized participatory modelling is to build a model that reflects peoples’ reality in a way that is useful to address and act on complex real-world problems. It is thus implied that participants construct evidence of their own reality and become the sole architects of collective knowledge, rather than serving as a source of parboiled data to cook in computational models. Such a positivist view of people leads to a different design of the research process, which may be agent-based, but not human-centered.
Basic elements of a research process which is human-centered, rather than agent-based, are that the community of stakeholders actually represent a community or, at the least, are given the opportunity to become a community in a repeated process of thinking and learning together; and, that the initial problem situation which becomes a question for research is defined by that community itself.
There are a number of strong examples of the constructive application of such an approach, e.g., Hovmund (2014) who have applied community-based system dynamics. Different types of group modelling can be characterized in that approach according to
-
who is defining the initial problem (the researchers or the community),
-
whether a structured or unstructured group modelling process is followed,
-
whether an informal causal map or a formal computer simulation model is built,
-
whether to start a group exercise open-ended with a “blank slate” or with an initial concept model. The main concern with using an initial structure, is that it may bias participants and create a strong framing effect.
Making the right choice for different group modelling approaches depends on how familiar the community is with group modelling and how literate group members are in the use of modelling language and tools. New communities will usually start with a “blank slate” approach to discover what their common issues are and how to ground problems in local language. Later as community members become more familiar with group modelling, they can make use of previously built models.
Making the right choice about methods applied is essential. Aaltonen and Sanders (2006)[iii] have proposed a framework which can serve as constructive guide for identifying the general epistemological and ontological assumption behind certain types of methods. As methodological plurality and mixing participatory and mathematical approaches is common place and often referred to as “participatory washing”, the framework is useful to bridge the gap between claim and reality of the participatory nature of methods, by the degree to which initial system conditions are identified and represented (Figure).
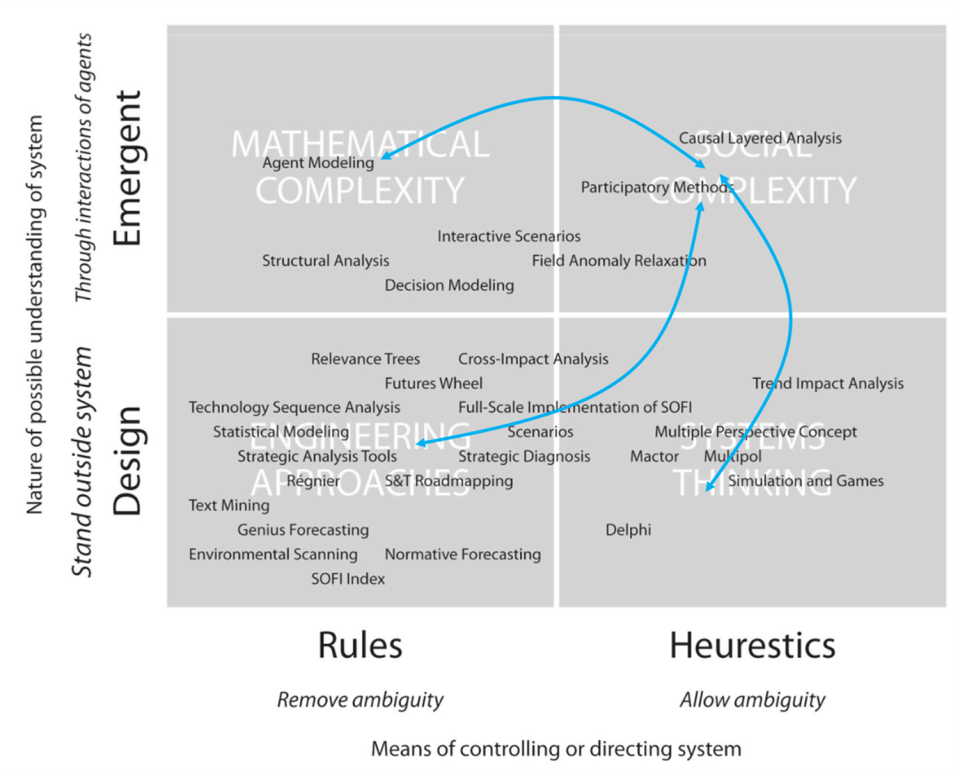
Engaging communities is fundamentally about building a shared language and community of practice, as no insights can be communicated and implemented without a shared language and understanding of a model within a community. In community-based modelling, “the model” begins with the issues that the community identify, not with the scientists’ preconception of what the problem is. Building a meaningful relationship with communities (without having to motivate them to participate by financial incentives) and enabling them to build the capacity they need to cope with complex problems – those are some important elements of a human-centered systems approach.
The Design Justice Network has created a set of ten principles that “rethinks design processes, centers people who are normally marginalized by design, and uses collaborative, creative practices to address the deepest challenges our communities face.” While these principles are aimed at the Design industry, they can be applied to research to ensure that any modeling is more accurate, holistic, and authentically based in realities of a community. For example, Principle 6 states: We believe that everyone is an expert based on their own lived experience, and that we all have unique and brilliant contributions to bring to a design process. And Principle 10 states: Before seeking new design solutions, we look for what is already working at the community level. We honor and uplift traditional, indigenous, and local knowledge and practices. These principles echoe the post-normal science approach, in particular the importance of an extended peer community and the idea of participation as justice.
If progress is to be made in participatory computational modelling, more efforts need to be made in communicating between social scientists, communities and computer scientists, about epistemology, methods and ethics of participatory modelling to ensure that communities are represented accurately, empowered to highest degree possible on solutions that will directly impact their lives, and to mitigate risk and reduce potential harm or unintended consequences.
[i] Lawrence, Roderick J. Co-Benefits of Transdisciplinary Planning for Healthy Cities, Urban Planning 2022 (7): 4 Healthy Cities: Effective Urban Planning Approaches to a Changing World, doi.org/10.17645/up.v7i4.5674
[ii] Lawrence, R.J., Gatzweiler, F.W. Wanted: a Transdisciplinary Knowledge Domain for Urban Health. J Urban Health 94, 592–596 (2017). doi.org/10.1007/s11524-017-0182-x
[iii] Aaltonen, M. and Irene Sanders, T. (2006), “Identifying systems’ new initial conditions as influence points for the future”, Foresight, Vol. 8 No. 3, pp. 28-35. doi.org/10.1108/14636680610668054
Suggested citation: Gatzweiler Franz, Antonaccio Cara and Chokdee Rutirasiri., "A Constructive Approach to Participatory Computerised Modelling," UNU Macau (blog), 2023-04-21, 2023, https://unu.edu/macau/blog-post/constructive-approach-participatory-computerised-modelling.