Seasonal climate forecasts are essential for decision-making in agriculture, energy, and disaster management but often suffer from spatially and temporally structured biases that reduce reliability. These biases, while challenging, offer opportunities for improving predictions, particularly for temperature extremes. Traditional bias correction methods like Quantile Mapping and Linear Scaling are limited by stationarity assumptions and their inability to capture complex spatiotemporal patterns. In this Science Talk, Dr. Rendani Mbuvha will introduce Season-Net, a hybrid deep learning framework combining U-Net and ConvLSTM architectures. Season-Net corrects biases in seasonal daily temperature forecasts from the UK Met Office and Météo-France by learning season-specific spatial and temporal dependencies. Trained with an extreme-aware, quantile-based loss function, it prioritizes accurate prediction of distribution tails, enhancing performance for extreme events. Evaluations across North America and Africa show Season-Net outperforms traditional methods in deterministic (RMSE, Kendall’s Tau) and probabilistic (Brier Skill Score, CRPSS) metrics, with significant improvements in predicting warm and cold spells. These results demonstrate Season-Net’s potential to advance seasonal forecast postprocessing, offering high-accuracy, impact-relevant predictions for climate-sensitive applications.
Speaker
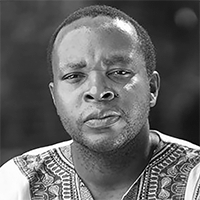
Research Fellow, Artificial Intelligence and Applied Statistics